教师发展中心“名师讲堂”活动特别邀请香港中文大学(深圳)的张纵辉副教授来校作学术交流,具体安排如下,欢迎广大师生参加。
一、主 题:z-SignFedAvg: A Unified Stochastic Sign-based Compression for Federated Learning
二、时 间:2023年5月15日(周一)10:00-12:10
三、地 点:科研楼C区218
四、主讲人:香港中文大学(深圳) 张纵辉 副教授
五、主持人:信息与通信工程学院 林静然 教授
六、主讲内容:
Federated Learning (FL) is a promising privacy-preserving distributed learning paradigm but suffers from high communication cost when training large-scale machine learning models. Sign-based methods, such as SignSGD, have been proposed as a biased gradient compression technique for reducing the communication cost. However, sign-based algorithms could diverge under heterogeneous data, which thus motivated the development of advanced techniques, such as the error-feedback method and stochastic sign-based compression, to fix this issue. Nevertheless, these methods still suffer from slower convergence rates. Besides, none of them allows multiple local SGD updates like FedAvg. In this paper, we propose a novel noisy perturbation scheme with a general symmetric noise distribution for sign-based compression, which not only allows one to flexibly control the tradeoff between gradient bias and convergence performance, but also provides a unified viewpoint to existing stochastic sign-based methods. More importantly, we propose the very first sign-based FedAvg algorithm (z-SignFedAvg). Theoretically, we show that z-SignFedAvg achieves a faster convergence rate than existing sign-based methods and, under the uniformly distributed noise, can enjoy the same convergence rate as its uncompressed counterpart. Extensive experiments are conducted to demonstrate that the z-SignFedAvg can achieve competitive empirical performance on real datasets.
七、嘉宾简介:
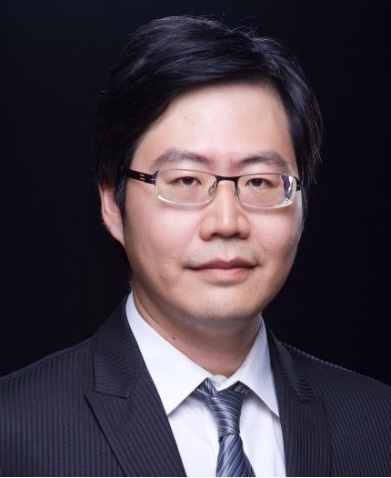
张纵辉,国家高层次青年人才,IEEE Fellow,现为港中文大学(深圳)理工学院副教授、助理院长(主管教育)、广东省大数据计算基础理论与方法重点实验室副主任、深圳市大数据研究院研究员。担任国际信号处理顶级期刊IEEE Trans. Signal Processing资深编委,网络信号处理顶级期刊IEEE Trans. Signal and Information Processing over Networks、IEEE Open Journal of Signal Processing编委,IEEE信号处理协会(SPS)通信网络信号处理技术委员会委员、感知通信一体化工作组发起人与首届主席和IEEE SPS董事会亚太区独立主席。
张纵辉教授专注于面向无线通信、机器学习的关键信号处理和优化方法的基础研究,已发表IEEE国际顶级期刊/会议论文160余篇。2015年获IEEE通信学会亚太区杰出青年学者奖;2018年和2021年两次获得IEEE信号处理学会(SPS)最佳论文奖;获得香港中文大学(深圳)理工学院首届卓越科研奖。近年来主持和参与包括国家自然科学基金重点项目、面上项目、广东省重点项目、深圳市杰出青年项目以及华为、中兴等企业的横向项目 10 余项。其中“分布式基带架构的新型信道估计算法”获得华为2022年技术成果转化二等奖。
八、主办单位:教师发展中心
承办单位:信息与通信工程学院