由信息与通信工程学院主办的“信通论坛”本次邀请到南洋理工大学蒋旭东教授,与我校师生共同探讨Sematic Scene Image Segmentation by Deep Machine Learning。具体安排如下,欢迎感兴趣的师生参加。
一、主 题:Sematic Scene Image Segmentation by Deep Machine Learning
二、主讲人:新加坡南洋理工大学 蒋旭东教授
三、时 间:2019年06月19日(周三)15:00-17:00
四、地 点:清水河校区科研楼B108
五、主持人:信息与通信工程学院 朱策教授
六、内容简介:
Scene image segmentation is a challenging task as it need classify every pixel in the image. It is crucial to exploit discriminative context and aggregate multi-scale features to achieve better segmentation. Context is essential for semantic segmentation. Due to the diverse shapes of objects and their complex layout in various scene images, the spatial scales and shapes of contexts for different objects have very large variation. It is thus ineffective or inefficient to aggregate various context information from a predefined fixed region. In this talk, I will first present a novel context contrasted local feature that not only leverages the informative context but also spotlights the local information in contrast to the context. The proposed context contrasted local feature greatly improves the parsing performance, especially for inconspicuous objects and background stuff. Furthermore, I will present a scheme of gated sum to selectively aggregate multi-scale features for each spatial position. The gates in this scheme control the information flow of different scale features. Their values are generated from the testing image by the proposed network learnt from the training data so that they are adaptive not only to the training data, but also to the specific testing image. Finally, I will present a scale- and shape-variant semantic mask for each pixel to confine its contextual region. To this end, a novel paired convolution is proposed to infer the semantic correlation of the pair and based on that to generate a shape mask. Using the inferred spatial scope of the contextual region, a shape-variant convolution is controlled by the shape mask that varies with the appearance of input. In this way, the proposed network aggregates the context information of a pixel from its semantic-correlated region instead of a predefined fixed region. In addition, this work also proposes a labeling denoising model to reduce wrong predictions caused by the noisy low-level features. This talk is based on two papers: H. Ding, X. Jiang, et al, “Context contrasted feature and gated multi-scale aggregation for scene segmentation,” CVPR’2018 Oral, and H. Ding, X. Jiang, et al, “Semantic Correlation Promoted Shape-Variant Context for Segmentation,” CVPR’2019 Oral.。
七、主讲人简介:
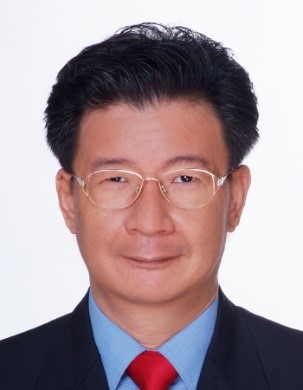
Xudong Jiang received the B.Eng. and M.Eng. degrees from the University of Electronic Science and Technology of China (UESTC), Chengdu, China, respectively, and the Ph.D. degree from Helmut Schmidt University, Hamburg, Germany, all in electrical engineering. He was a Lecturer with UESTC, where he received two Science and Technology Awards from the Ministry for Electronic Industry of China. From 1998 to 2004, he was with the Institute for Infocomm Research, A*STAR, Singapore, as a Lead Scientist, and the Head of the Biometrics Laboratory, where he developed a system that achieved the most efficiency and the second most accuracy at the International Fingerprint Verification Competition in 2000. He joined Nanyang Technological University (NTU), Singapore, as a Faculty Member, in 2004, where he has also served as the Director of the Centre for Information Security from 2005 to 2011. He is currently a tenured Assoc Professor with the School of EEE, NTU. He holds 7 patents and has authored over 150 papers with over 30 papers in the IEEE journals, including 9 papers in IEEE T-IP, 6 papers in IEEE T-PAMI, and 3 papers in IEEE T-SP. Two of his first authored papers (both in IEEE T-PAMI) have been listed as top 1% highly cited papers in the academic field of Engineering by Essential Science Indicators. His H-index is 30 on Web-of-Science, 34 on Scopus and 41 on Google Scholar. His research interests include signal/image processing, pattern recognition, computer vision, machine learning, and biometrics. He has been an IFS Technical Committee Member of the IEEE Signal Processing Society, and he has served as Associate Editors for the IEEE Transactions on Image Processing, IEEE Signal Processing Letters, and IET Biometrics.
八、主办单位:发展规划与学科建设处
承办单位:信息与通信工程学院