On February 24th, the official results of the 2020 IEEE International Conference on Computer Vision and Pattern Recognition (CVPR) were announced. TanWANG, a 2016 undergraduate student from the School of Information and Communication Engineering, under the guidance of Prof. Hanwang Zhang at Nanyang Technological University and with sponsorship from Alibaba DAMO Academy, had his paper titled "Visual Commonsense R-CNN" accepted by CVPR 2020 as the first author. He becomes the first undergraduate student from our university to publish as the first author at CVPR.
CVPR is one of the world's top three conferences in the field of computer vision, and this edition received over 10,000 submissions from around the world. Of these, 6,656 were deemed valid, and the final acceptance rate was 22%, the lowest in the past decade. The conference is scheduled to be held in Washington, USA, from June 16-19.
The paper, "Visual Commonsense R-CNN," addresses issues with existing Up-Down features used in Vision & Language tasks, such as significant bias and the lack of relationships between objects. Taking a causal inference perspective and leveraging Judea Pearl's "Do" operator and the backdoor adjustment algorithm proposed in 2009, the paper intervenes in real-world scenes by combining existing object detection frameworks. This intervention can be conceptually understood as "Borrow & Put":
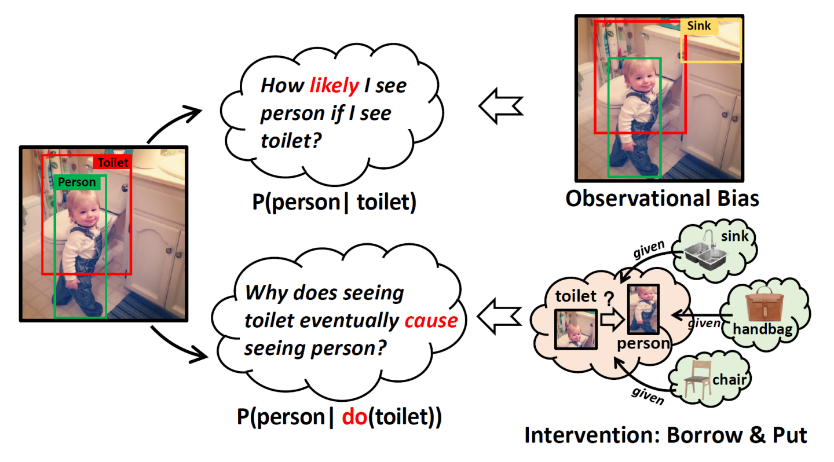
Figure 1: Comparison with traditional Bayesian conditional probabilities
Constructing a dictionary to "borrow" objects that are widely present in other images to the current image.
Then "putting" the borrowed objects around X, Y and contrasting with X, Y. For example, in the illustration, moving objects like sinks, handbags, chairs, etc., around the toilet and person. The post-intervention values are calculated using the backdoor adjustment formula.
The paper finally employs a self-supervised learning approach to learn better representations of local objects in images, termed "visual commonsense features." The framework diagram is shown in Figure 2. For detailed calculations, refer to the article (see the link at the end).
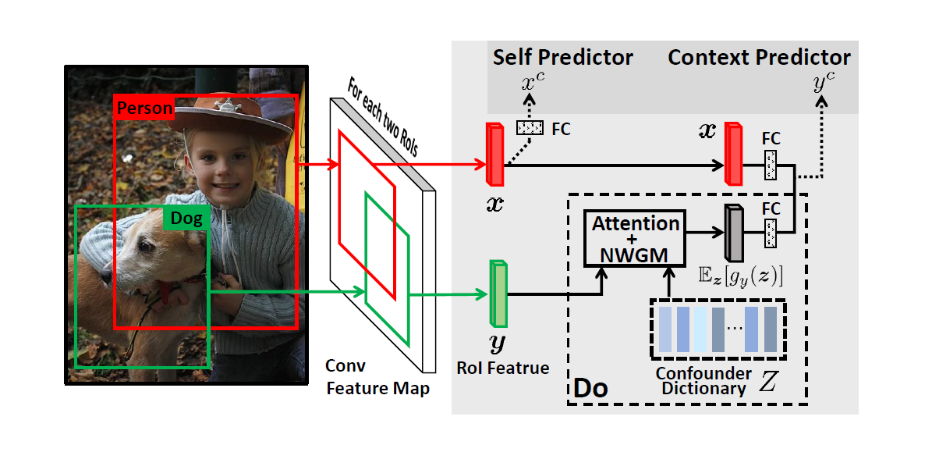
Figure 2: Frame structure diagram of visual common sense feature extraction
The authors validated the learned features in three major Vision & Language downstream tasks, achieving the best results in all. Particularly in the image captioning task, the paper achieved an improvement of nearly 2 percentage points in Cider compared to the original.
Tan WANG interned at Nanyang Technological University in Singapore in July 2019, and the publication of this paper is the result of extensive effort over time. This research is closely related to the currently highly regarded area of self-supervised learning. The challenge in self-supervised learning lies in the lack of direct evaluation metrics, requiring a considerable amount of experimentation to validate the algorithm's effectiveness. It is hoped that this paper will bring more value to the academic community.
Tan WANG's Achievements:
During his time at the university, Tan WANG received the National Scholarship and Tang Lixin Scholarship. With a weighted average score of 92.8 and a GPA of 3.99, he consistently ranked 1st out of 450 in comprehensive rankings in the first two years of his major. Among the 67 courses he took, 62 scored above 90, earning him the title of Provincial Outstanding Graduate. In November 2019, a paper he authored as the first author, titled "Matching Images and Text with Multi-modal Tensor Fusion and Re-ranking," was accepted as an Oral (main session presentation) paper at the 27th ACM International Conference on Multimedia. He has now received a fully-funded Ph.D. offer in Computer Vision at the Hong Kong Chinese University.
Reference Links:
Paper Link: https://arxiv.org/abs/2002.12204)
Detailed algorithm code can be found in Tan WANG's GitHub repo. Feel free tovisit: https://github.com/Wangt-CN/VC-R-CNN
- Zhihu Popular Science Article: https://zhuanlan.zhihu.com/p/111306353