Recently, the European Conference on Computer Vision (ECCV) 2020 announced its list of accepted papers, and SICE Intelligent Vision Information Processing team’s first-year graduate student, Longrong YANG, had his paper titled "Learning with Noisy Class Label for Instance Segmentation" successfully accepted. Longrong YANG is the first author of the paper, with Professor Hongliang LI as the corresponding author, and UESTC as the sole affiliation. Additionally, Yuyang QIAN, a 2016 undergraduate student from SICE, authored a paper titled "Thinking in Frequency: Face Forgery Detection by Mining Frequency-aware Clues," during his research internship at the Sensetime Research Institute, which was also accepted at the conference. Yuyang QIAN is the first author, and UESTC is the first affiliation.
ECCV is held biennially and is one of the three top conferences in the field of computer vision, alongside CVPR and ICCV, garnering widespread attention from the global academic and industrial communities.
Based on the fact that the foreground-background information provided by the noisy category labels is always correct, Longrong YANG designed a new combinatorial loss to make full use of the noisy category labels in the subtask of multi-instance segmentation foreground-background classification. Instance segmentation is a basic and challenging computer vision research topic, which includes two sub-tasks: foreground-background classification and foreground-instance classification.The paper focuses on the importance of data in instance segmentation. However, the ambiguity of the category itself or limitations in annotator experience can lead to mislabeled class labels, significantly degrading the model's accuracy. Moreover, noise-robust symmetric loss proposed in classification tasks severely deteriorates the accuracy of foreground-background classification in instance segmentation.
Specifically, the paper divides samples within a batch into four categories: negative samples (NEG), pseudo-negative samples (PSN), potential noise samples (POS), and other samples (OS). Different losses are employed for different sample types. The proposed method demonstrates stable improvements in model accuracy across multiple databases and various noise sample settings, consistently outperforming existing methods.
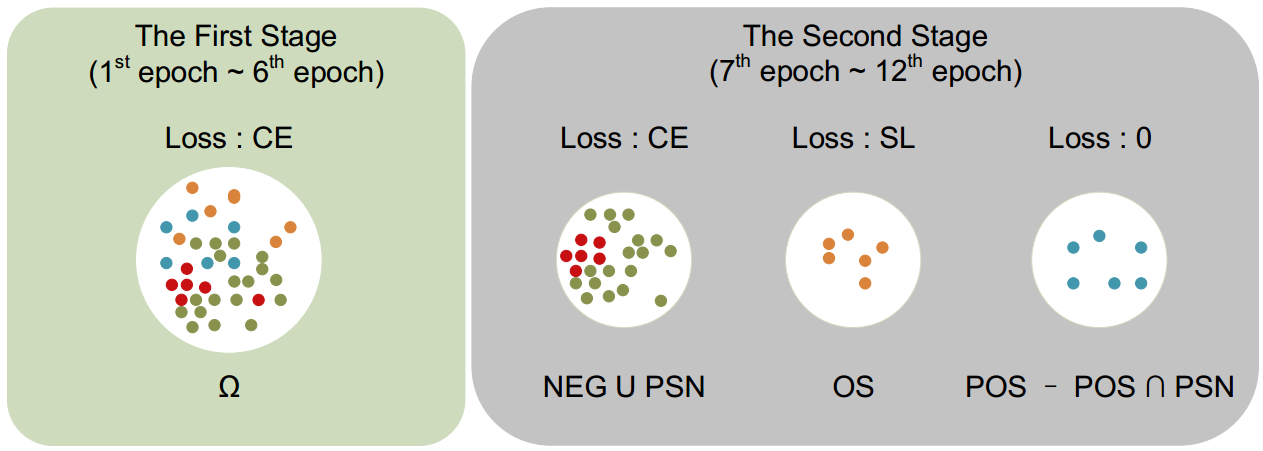
Figure 1: Losses used by different samples within each batch. In the second stage of training, negative and pseudo-negative samples use cross-entropy loss to make full use of the pre-background information with the correct label of the noisy category
Paper Details:https://github.com/longrongyang/LNCIS
In recent years, with the advancement of artificial intelligence, particularly deep learning, Face Forgery and Deepfake (A portmanteau of deep learning and fake, which is the use of machine learning techniques to "swap faces" and fake images and videos of someone) technologies have become more mature, capable of generating and manipulating more realistic faces. If maliciously exploited, the consequences can range from infringing on others' portrait rights in a humorous context to impacting the image of political figures.
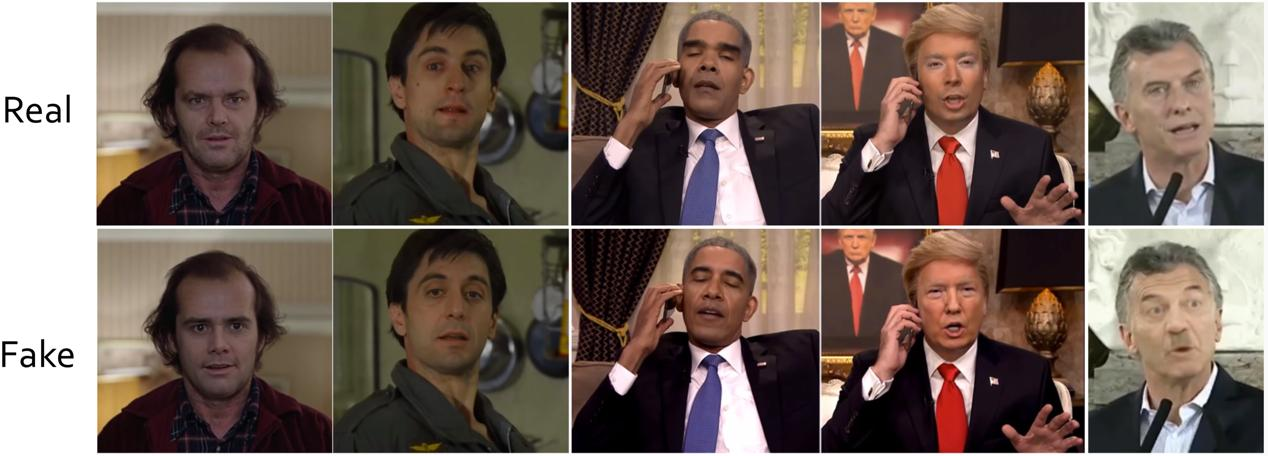
Figure 2: Face Forgery and deepfake techniques can fake realistic images, the first is the original real image, and the second is a fake image generated by machine learning technology (Source: YouTube).
To more accurately identify these Deepfake images and videos for detection, Yuyang QIAN's work proposes the innovative Frequency in Face Forgery Network (F3-Net). Unlike previous techniques using spatial domain features (such as RGB, HSV features, etc.), F3-Net focuses more on the frequency domain features of images. This is because small-scale artificial manipulation traces in low-resolution images, although challenging to observe in the RGB space, can be easily identified in the frequency domain. F3-Net accurately identifies small-scale manipulation traces in low-resolution images by exploring multiple frequency domain features.
Specifically, F3-Net first extracts two types of frequency domain features: Frequency-Aware Decomposition (FAD) and Local Frequency Statistics (LFS). It then designs the MixBlock module, which combines the two features using a cross-attention mechanism and jointly optimizes them to produce the final output. The network architecture is illustrated in Figure 3.
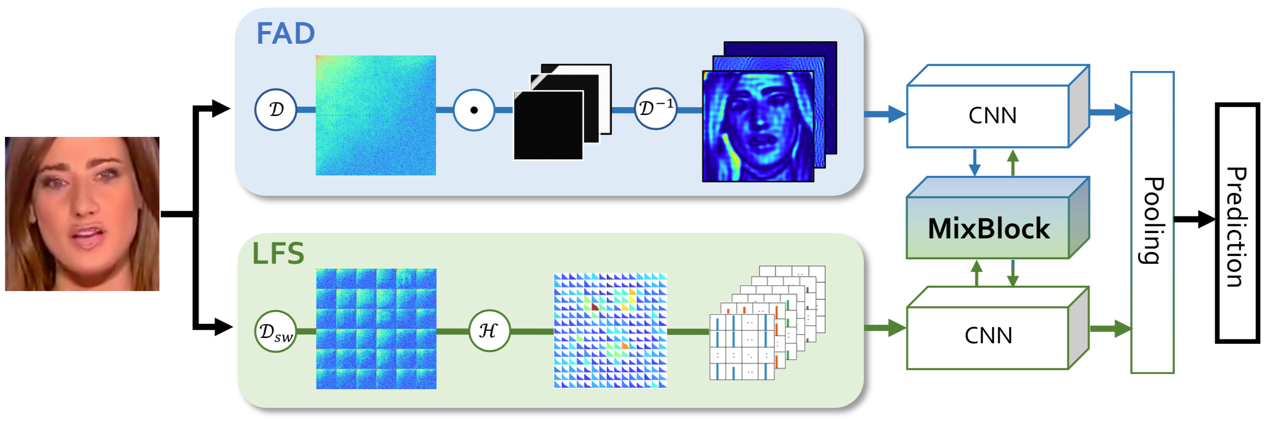
Figure 3: Network diagram of F3-Net
The two frequency domain features (FAD and LFS) extracted from the images by F3-Net are the innovation and core of this work. In the traditional method, people use a manually designed fixed filter to extract the frequency domain features, while FAD uses a learnable filter to adaptively decompose the image frequency domain features, which can more accurately find the forgery traces in different frequency band components. LFS extracts the local frequency domain statistics of the image, which is more sensitive to the anomaly in the details, and LFS uses the sliding window DCT technology to retain the structural information of the image, making it compatible with CNN networks.
Experimental results show that F3-Net achieves good results on the FaceForensics++ (FF++) dataset, and the recognition accuracy is about 4% better than that of the previous SOTA method. In particular, a large improvement has been achieved in compressed low-quality (LQ) image videos. By mining the frequency domain features, F3-Net can more accurately "visualize" images and videos that are difficult to distinguish.
Paper Link:https://arxiv.org/abs/2007.09355
Related Link:
Longrong YANG: Since joining Professor Hongliang LI's team in his senior year, Longrong YANG has participated in several research projects. He previously won first prize in the Intelligent Analysis of Remote Sensing Image competition hosted by the National Natural Science Foundation of China (NSFC). In recent years, the Intelligent Vision Information Processing team, led by Professor Hongliang LI, has actively encouraged students to integrate theory with practice, fostering a pragmatic learning style and comprehensive knowledge application skills, resulting in a series of excellent achievements.
Yuyang QIAN: With an average GPA of 3.98, Yuyang QIAN ranked in the top 5% of his major. He won a gold medal in the 2018 ACM CCPC China National College Student Programming Invitational. He received various awards, including the 2019 Honor SICE Annual Character Award, Excellent Student Scholarship, and Outstanding Undergraduate Thesis. Currently, he has been admitted without an exam to pursue graduate studies at Nanjing University's LAMDA Laboratory.